Our recent articles have looked at offloading computations to accelerators from Fortran programs:
- Accelerating LU Factorization Using Fortran, Intel® oneMKL, and OpenMP*
- Solving Heterogeneous Programming Challenges with Fortran and OpenMP
- Solving Linear Systems Using oneMKL and OpenMP Target Offloading
- Using Fortran DO CONCURRENT for Accelerator Offload
This article will take a closer look at the advantages and disadvantages of the Fortran DO CONCURRENT statement and the OpenMP target constructs with respect to accelerator offload.
The DO CONCURRENT construct was introduced in ISO Fortran 2008 to inform, or assert to, the compiler that the iterations of the DO CONCURRENT loop are independent and can be executed in any order. A DO CONCURRENT loop can be executed sequentially, in parallel, and can even use the OpenMP backend to offload DO CONCURRENT loops to accelerators. Accelerator offload capability was added to OpenMP version 4.0 in 2013. The OpenMP target directives allow the programmer to specify regions of code to execute on an accelerator as well as the data to be transferred between the host processor and the accelerator.
Both approaches to accelerator offload are portable to any system with standards-compliant compilers. DO CONCURRENT has the advantage of a concise, ISO Fortran syntax. However, ISO Fortran has some of the same limitations as ISO C++. (Editor’s note: See The Case for SYCL* for a review of ISO C++ limitations with respect to heterogeneous parallelism.) It has no concept of devices or disjoint memories, so there’s no standard way to transfer flow of control to an accelerator or to control host-device data transfer. OpenMP addresses these limitations. It’s not an ISO standard, but it’s an open industry standard with over 25 years of maturity. The OpenMP target directives are more verbose and require the programmer to describe the parallelism to the compiler, but they give fine control over host-device data transfer and allow aggregation of parallel regions for better efficiency, as we’ll see in the code examples below.
Our previous article, Using Fortran DO CONCURRENT for Accelerator Offload, applied a simple filter to binary images to illustrate edge detection. Here, we will use a more realistic edge detection algorithm for our comparison. We’ll implement the Sobel algorithm using Fortran DO CONCURRENT and OpenMP target directives. This algorithm applies horizontal and vertical filters to each pixel of the original image then looks for sharp changes in pixel intensity in the transformed image:
A before and after example is shown in Figure 1. The operation on each pixel is independent, so the algorithm is highly data parallel.
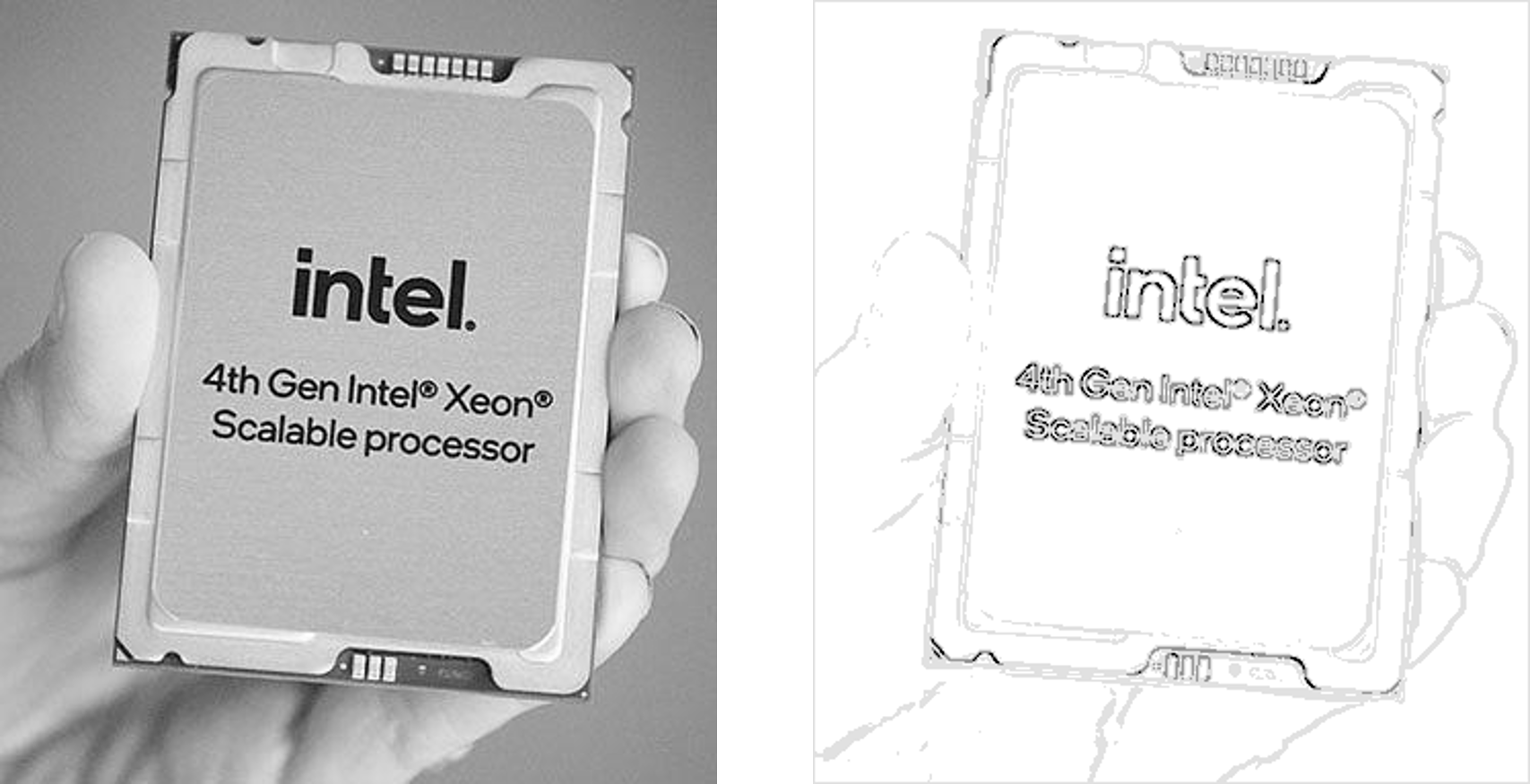
The Sobel algorithm is typically implemented in three dependent steps: image smoothing, edge detection, and edge highlighting. (Editor’s note: Readers may know from my previous articles that I’m partial to multistep algorithms because they can highlight synchronization and data dependencies better than simpler algorithms, e.g.: Accelerating the 2D Fourier Correlation Algorithm with ArrayFire and oneAPI.) These steps are easily coded using three Fortran DO CONCURRENT loops (Figure 2). Each loop has significant data parallelism depending on the size of the image. Our example assumes 2D images, but the algorithm can be extended to volumetric images.
The DO CONCURRENT construct is just another form of the DO construct. Even if this is your first time seeing DO CONCURRENT, it should be clear to most Fortran programmers that this example iterates over the columns and rows of the image like a familiar doubly nested DO loop. DO CONCURRENT loops can have predicates to mask some iterations, or additional clauses to define the scope of variables (e.g., the second loop in Figure 2 contains a reduction operation).
The data structure containing the pixel values has red, green, and blue channels (fields), but images are typically converted to grayscale prior to Sobel edge detection. This reduces computation because the channels are equivalent in a grayscale image. Therefore, we only have to operate on one channel in each step of our Sobel implementation. This doesn’t just reduce the amount of computation, however. It also reduces the amount of data that has to be transferred between host and device memories, as we shall see below.

The Intel® Fortran Compiler can offload DO CONCURRENT loops to an accelerator using the OpenMP backend. We use the following command to build our example program for accelerator offload using the OpenMP backend and ahead-of-time compilation for the “pvc” device (i.e., the Intel® Data Center GPU Max):
$ ifx ppm_image_io.F90 sobel_do_concurrent.F90 -o sobel_dc_gpu -qopenmp \ > -fopenmp-targets=spir64_gen -fopenmp-target-do-concurrent \ > -Xopenmp-target-backend “-device pvc”
The first source file (ppm_image_io.F90) is a utility module to handle image I/O. The second source file (sobel_do_concurrent.F90) contains the code in Figure 2. We’ll run the executable on an 8K (7,680 x 8,404) resolution image (64,542,720 pixels x 4 bytes/pixel = 258,170,880 bytes) in PPM format:
We’ve color-coded the red, green, and blue image channels being transferred between host (hst) and target (tgt) device memories. The three sections delineate the three DO CONCURRENT loops in Figure 2. (Fortran array descriptors, or dope vectors, of the arrays being mapped to the target device are not shown. Dope vectors are small, so this data movement can be ignored. Likewise, the 3 x 3 filter matrices are not shown either.) Host-device data transfer is being handled implicitly. This is convenient for the programmer, but not always efficient. For example, notice that the first DO CONCURRENT loop in Figure 2 only reads the blue channel and only writes the red channel. Therefore, the first DO CONCURRENT loop should only require one hst→tgt and one tgt→hst transfer. However, all three channels are being transferred to the device and back to the host.
That’s a lot of unnecessary data movement. Moving data between disjoint memories takes time and energy, so minimizing host-device data transfer is critical to heterogeneous parallel performance. Unfortunately, the ISO Fortran 2018 and forthcoming 2023 standards don’t provide language constructs to control data movement or to tell the runtime when data is read-only or write-only.
Fortunately, the OpenMP target offload API provides a way to aggregate the three steps of our Sobel implementation into a single target data mapping region so that data is only transferred when necessary (Figure 3).
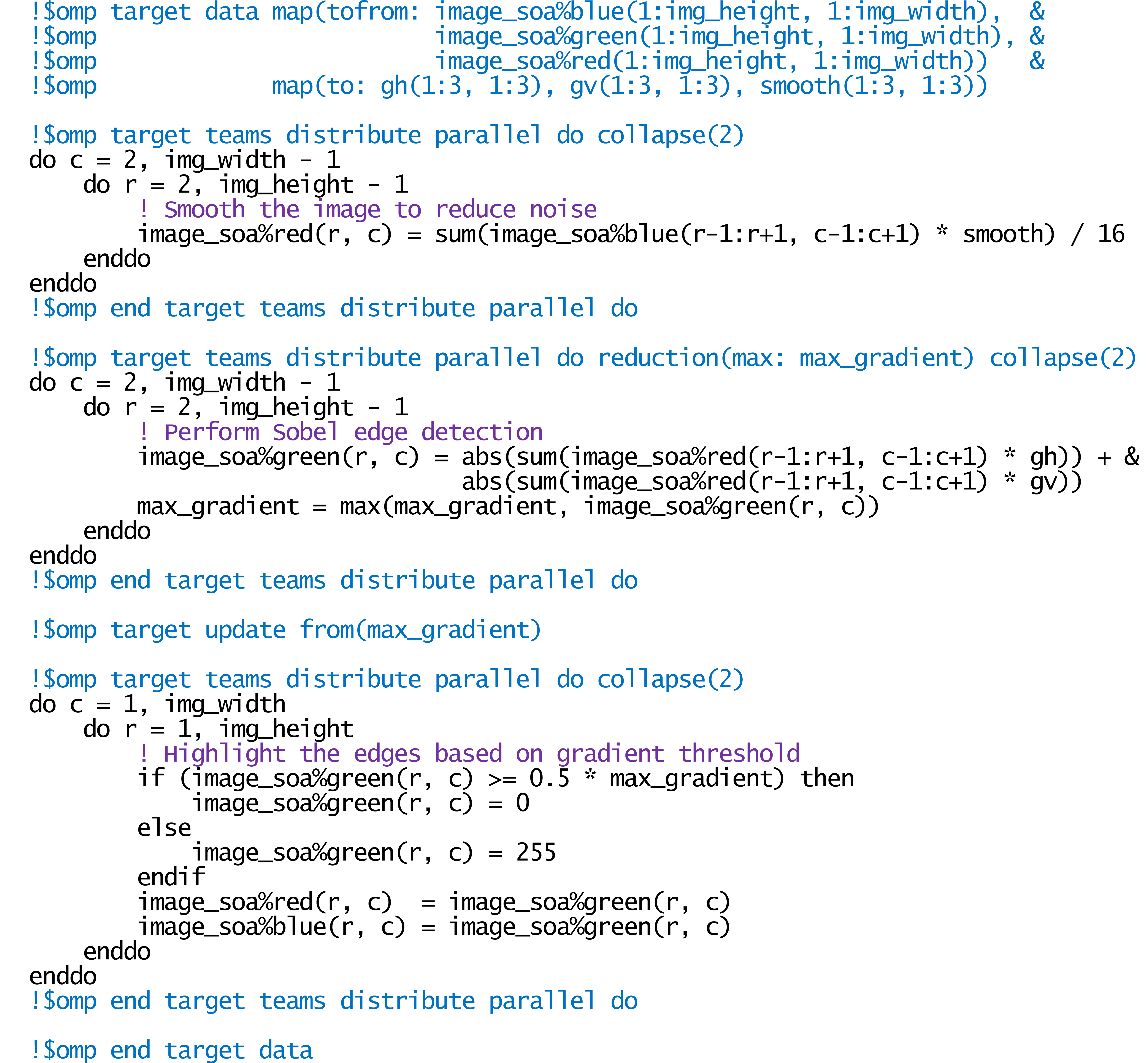
We compile and run the OpenMP implementation in Figure 3 as follows:
Once again, we’ve removed the small dope vectors and filter matrices and color-coded the red, green, and blue image channels being transferred between host (hst) and target (tgt) device memories. Notice that the channels are only copied once in each direction, which is significantly less than the DO CONCURRENT implementation. As you can see, OpenMP gives us finer control over data movement than is currently possible with ISO Fortran alone.
OpenMP target offload also gives better performance than DO CONCURRENT when computing Sobel edge detection on a large image (Table 1). The DO CONCURRENT (Figure 2) and OpenMP target (Figure 3) examples give equivalent performance (0.1 seconds) on the host CPU, but OpenMP target offload to the GPU gives the best performance overall (0.05 seconds). In fact, the unnecessary data movement in the DO CONCURRENT example hurts the GPU performance (0.18 seconds).
OpenMP | DO CONCURRENT | |
---|---|---|
Sequential | 0.37 | 0.37 |
Parallel (CPU) | 0.1 | 0.1 |
Parallel (GPU) | 0.05 | 0.18 |
Table 1. Comparing OpenMP* target and Fortran DO CONCURRENT performance for an 8K (7,680 x 8,404) resolution image (all times in seconds). The sequential baselines are the OpenMP (sobel_omp_target.F90) and DO CONCURRENT (sobel_do_concurrent.F90) examples compiled without OpenMP enabled. The CPU and GPU are Intel® Xeon® Platinum 8480+ and Intel® Data Center GPU Max 1100, respectively.
The DO CONCURRENT construct provides a convenient syntax to express implicitly parallel loops, but until ISO Fortran has a concept of disjoint memories, and a corresponding syntax to control host-device data transfer, it is best to combine Fortran and OpenMP for heterogeneous parallelism.
Our source code, along with a small test image, are available at sobel_example. You can experiment with Fortran DO CONCURRENT and OpenMP accelerator offload on the free Intel® Developer Cloud, which has the latest Intel® hardware and software.